Enhancing Time-Series Analysis with Multimodal Models
Unlocking the power of time-series data with multimodal models 🔗
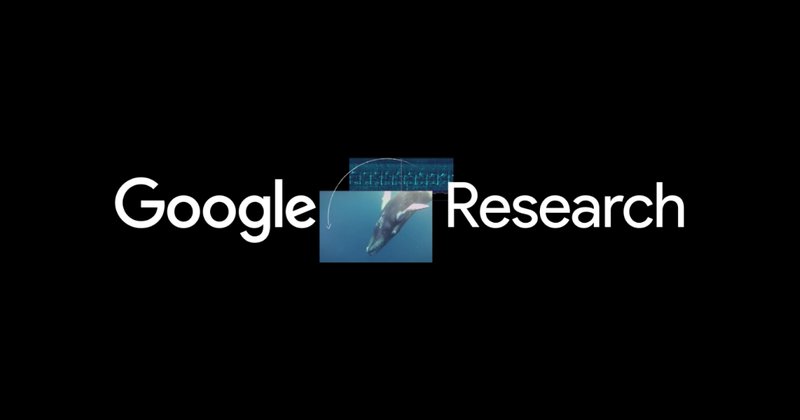
Multimodal models have shown significant improvements in understanding time-series data when presented as visual plots rather than numerical values. Research indicates that using plots can enhance performance in classification tasks, such as fall detection, by up to 120%. The study utilized both synthetic and real-world data, revealing that multimodal models, like Gemini Pro and GPT4o, excel at interpreting visual information. Plotting time-series data not only improves accuracy but also makes more efficient use of the models' context windows, lowering the computational costs involved. This research suggests a promising direction for leveraging multimodal capabilities to enhance the analysis of complex data across various fields, including healthcare.
What is the main finding of the research regarding multimodal models and time-series data?
The research found that multimodal models perform significantly better when analyzing time-series data presented as visual plots compared to numerical values, achieving performance improvements of up to 120% in classification tasks.
Which tasks were tested to evaluate the performance of the multimodal models?
The study tested the models on tasks such as fall detection using IMU data and recognizing physical activities, along with synthetic tasks designed to assess various reasoning capabilities.
How does using plots affect the computational efficiency of multimodal models?
Using plots allows multimodal models to make more efficient use of their context windows, as visual representations require fewer tokens than numeric strings, leading to potential cost savings in reasoning about the data.